Sheryl Swink1, Tulsi Kharel1, Dilip Kharel1, Angel Maresma1, Erick Haas2, Ron Porter2, Karl Czymmek1,2, and Quirine Ketterings1
1Cornell Nutrient Management Spear Program, 2Cazenovia Equipment Company, 3PRODAIRY
Introduction
Reliable yield maps allow farmers and farm consultants to analyze yields per field, within fields, across fields and across years. Yield maps can be used to develop yield stability zones, or to identify reason(s) for low/high yielding areas by overlaying them with other geospatially tagged data such as elevation maps, soil series maps, etc. For reliable data, pre-harvest calibration of yield monitors and sensors should be followed up by careful operation in the field and proper post-harvest data cleaning in the office (Figure 1). This article presents best practices (pre-harvest, in-field, and post-harvest) that minimize yield monitor data errors and noise, reduce loss of data, and speed up data cleaning.
Pre-Harvest
- Field naming. Develop a simple and consistent set of field IDs or names for each farm. Make sure all operators know and use the correct field identification. Using numbers eliminates spelling errors. Inconsistency in a field’s name from year to year results in extra, time consuming, post-harvest data clean-up.
- Field boundaries. Establish and load geo-spatially fixed/frozen field boundary files into the Yield Monitor prior to harvesting. This will assist in maintaining the accuracy of field IDs. Preloading fixed field boundaries facilitates assignment of harvest data to the correct fields as the harvester moves from field to field. Follow the procedures in your Yield Monitor manual to load boundary files before harvest begins.
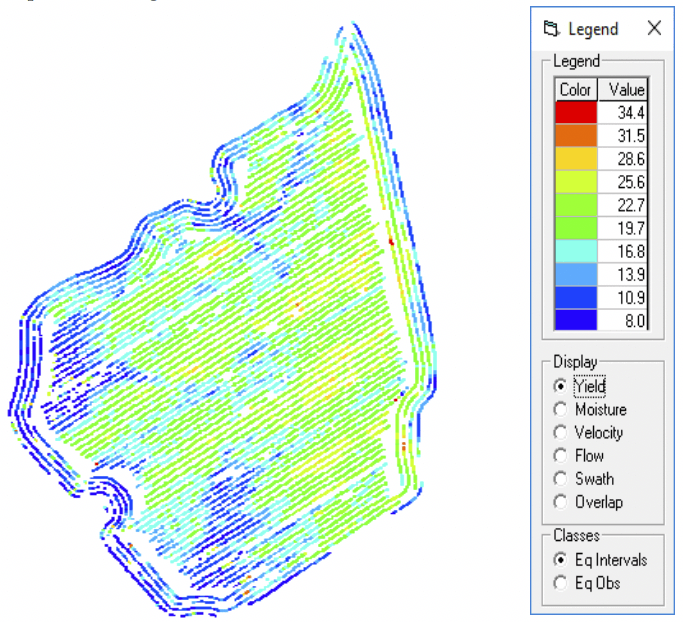
In-Field
- Calibrate. Calibration using accurate scale weights or a grain cart with load sensors will increase accuracy. When calibrating, harvest as you would normally do in average crop areas in the field (include variability in the field, not just the best part). Re-calibrate the yield monitor often – for each crop or even variety that is being harvested, and for significant changes in crop conditions (very dry to very wet). Check and zero the mass flow sensor every morning so that the sensor identifies crop flow accurately. Clean the lens of the moisture sensor and inspect for damage daily.
- Field name/ID. Check to be sure correct field name/ID is entered or displayed before harvester enters a new field. Avoid inventing field names “on the fly.” Carefully check spelling if manually entering a field ID while harvesting. Misspelled or variations in field names from season to season make it difficult to match field data files across years for yield comparisons and within-field variability analysis. Proper field naming will ensure that yield data are assigned to correct field files.
- Harvest speed. Maintain a steady harvest speed within the calibration range for your system. Yield data recorded outside of the calibration range will be less accurate (irregular and/or very slow or high velocities over parts of the field result in yield calculations errors).
- Header height. Be sure the monitor logs a start and stop for each directional pass across the field to ensure data and yield area are logged properly. In most cases, the operator must lift the header beyond a set height to trigger the “stop logging” signal when exiting a pass or turning in the field. For some equipment, material flow can also be used to log the end of passes when the header is not raised for turning or for driving in the field without harvesting. Correctly logged field passes expedite trimming of unrepresentative start and end pass data points (ramping effect) during the cleaning process and proper shifting of data when correcting for flow and/or moisture delays relative to GPS location.
- Swath width. Be sure the recorded swath width is the actual width harvested. If swath width is not recorded properly, the harvested area calculated is wrong and so is the yield value. If the GPS system of the yield monitor has a large positional error (e.g. WAAS), turn off the auto swath adjustment and manually enter the default swath/chopper width. When harvesting less than the default chopper width without auto-swath, manually adjust swath width of the pass in the yield monitor to avoid erroneous yield calculations.
- Short rows. For long, narrow fields, plant and harvest rows the length of the field rather than the width if practical and consistent with soil conservation and other farm objectives. Short harvest passes distort yield data due to ramping velocity and flow impacts at the beginning and end of a pass, leaving few or no accurate data points in very short passes.
- Multiple combines/choppers in the field. If using more than one combine or chopper on a field, harvest a discrete section of the field with each one rather than mixing their passes across the whole field. Differences between operators, equipment and sensors result in different flow and moisture delays. These factors, if interlaced across the field, make it difficult to properly clean data.
Post-Harvest
Do not risk losing the season’s data by just leaving it on your monitor or relying on the cloud to save it. Download the raw yield monitor data files periodically during the season. The data cleaning protocol requires raw data to be transferred into Ag Leader format. Save the original files, backing them up on thumb drives and on your computer.
In Summary
Reliable data are essential for making the right decisions in field management. Mitigating errors at the source reduces the amount of data loss when filtering out noise during the post-harvest data cleaning process. The accuracy of yield data depends not only on proper calibration of yield monitoring equipment prior to and during harvest, but also on operation in the field and post-harvest data cleaning. Data become more reliable and the data cleaning process can be accelerated with implementation of the pre-harvest, in-field, and post-harvest practices described in this article.
Acknowledgements
This work was co-sponsored by the United States Department of Agriculture, National Institute of Food and Agriculture, Agriculture and Food Research Initiative Bioenergy, Natural Resources and Environment program, grants from the Northern New York Agricultural Development Program (NNYADP), New York Farm Viability Institute, New York Corn Growers Association, and Federal Formula Funds. For questions about these results, contact Quirine M. Ketterings at 607-255-3061 or qmk2@cornell.edu, and/or visit the Cornell Nutrient Management Spear Program website at: http://nmsp.cals.cornell.edu/.